INFERRING AND REPLICATING ACTIVITY SELECTION AND SCHEDULING BEHAVIOR OF INDIVIDUALS
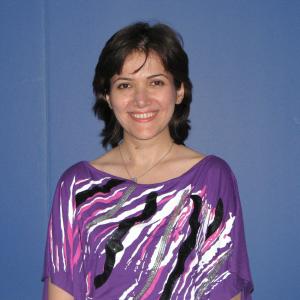
Understanding the choices that each individual in the population makes regarding daily plans and activity participation behavior is crucial to forecasting spatial-temporal travel demand in the region. The focus of this dissertation is developing a comprehensive mathematical/statistical framework to infer and replicate travel behavior of individuals in terms of their socio-demographic profiles. The framework comprises series of distinct modules that employ statistical segmentation, Bayesian econometrics, data mining, and optimization techniques to predict individuals’ activity types, activity frequencies, and the travel linkages that make them possible.
The key advantages of the model are: first; providing the likely content of activity agenda as part of the inference procedure, second; integrating transportation network topology within activity scheduling step, and third; integrating modal components. As part of the dissertation, a Graphical User Interface was developed for practical application of the model in transportation agencies. The data used for the analysis is the California Household Travel Survey data, 2000-2001. Testing the entire modeling system on an out-of-sample population—15% of the entire sample— shows that the model is able to predict on average 80.3% of daily activities of individuals correctly.