TRIP CHAINING COMPLEXITY, VARIABILITY, AND PATTERN USING LONGITUDINAL GPS DATA
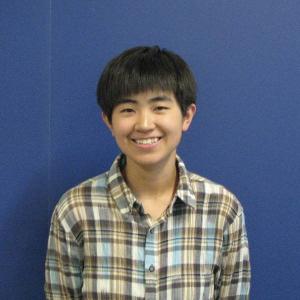
Trip chaining is a common phenomenon generally known as linking multiple activities and trips in one travel process. A good understanding about trip chaining complexity is important for travel demand model development and for transportation policy design. However, most of the existing studies on trip chaining limit the complexity classification scheme on number of trips chained and neglect other dimensions that also elevate the degree of complexity. The purpose of this study is to develop a new approach, Tour Complexity Index (TCI), that integrates the multi-dimensional nature of trip chaining into the complexity assessment.
The study contains three analysis components. The first component introduces the TCI approach as a trip chaining complexity measure that not only considers number of trips chained but also includes the spatial relationship across destinations, the route arrangement, and the urban environment of the destinations. By comparing descriptive statistics and generalized linear model results from TCI approach with those from traditional approach, we find that the TCI approach offers more information regarding trip chaining and mode choice. The application of TCI is further demonstrated in the following components. The second component investigates the intrapersonal daily and weekly travel variability with travel characterized by TCI and mode choice. The result reinforces an argument in current literature that the common single-day travel survey may produce biased estimation due to the day-to-day variance in travel behavior. Result also finds that proximity to a new transit service from place of residence is connected with a decline in variability. The third component explores a framework for travel pattern recognition where pattern is characterized by TCI as well. The discrepancy analysis which is a generalized analysis of variance (ANOVA) method is applied to associate individual characteristics with travel pattern. In addition, both components use Sequential Alignment Method (SAM) for travel pattern representation. The TCI approach and proposed analysis frameworks are validated using the longitudinal GPS trajectory data collected between 2011 and 2013 at west Los Angeles area for Expo Study.