Street network sprawl and autonomous vehicles
UC ITS Statewide Transportation Research Program (STRP)
University of California Institute of Transportation Studies' Resilient and Innovative Mobility Initiative (RIMI)
NSF Smart and Connected Communities Project (NSF S&CC)
ITS Graduate Student Association
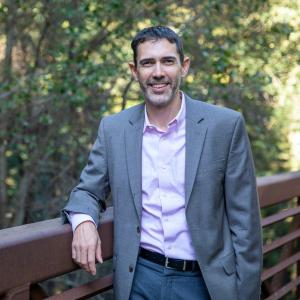
Urban Planning
Luskin School of Public Affairs
UCLA
Disconnected urban street network – “street-network sprawl” – are strongly associated with increased vehicle travel, energy use and CO2 emissions. Moreover, since street layouts are essentially permanent, today’s choices on the connectivity of streets will restrict future resilience and lock in car dependence for a century or more. Using data on all 46 million km of mapped streets worldwide and a satellite-derived time series of urbanization, I present the first systematic and globally commensurable measures of street-network sprawl.
I then discuss an emerging transportation technology. I highlight the autonomous vehicle parking problem: because such vehicles can avoid parking charges, they could undermine a key plank of transportation demand management and nearly double vehicle travel in city centers. Moreover, autonomous vehicles may find cruising around to be a lower cost option while waiting for their next rider, exacerbating congestion and environmental impacts.
Mr. Adam Millard-Ball is associate professor of Urban Planning at the UCLA Luskin School of Public Affairs. His research and teaching are about transportation, the environment, and urban data science. Trained as an economist, a geographer, and an urban planner, he analyzes the environmental consequences of transportation and land-use decisions, and the effectiveness of policies to reduce greenhouse gas emissions. His research uses large-scale geospatial data analysis as well as econometric and qualitative methods.