Advances in traffic surveillance technology can provide more complete and intelligent data from detectors. This dissertation describes an improved method of freeway performance measurement that integrates multi-sensor data fusion with a vehicle-monitoring algorithm capable of identifying the same vehicle/s at different locations. To obtain a more robust and effective data set for vehicle monitoring, data fusion from two state–of–the-art traffic detectors — loop detectors and video detectors — was introduced. Investigations and development of a new algorithm for data fusion and real-time vehicle monitoring – TRASURF (TRAffic SURveillance and perFormance) were also described. The algorithm’s development was based on an examination of feature vector extraction from each advanced traffic sensor, data fusion across multiple technologies and analysis of sensor performance. A real-world data set from one section of the I-405 freeway was applied to develop and evaluate the algorithm for a single freeway section. Based on extensive analysis of these field data, the PARAMICS (PARAllel MICroscopic Simulation) model was used to generate simulated fused data. This simulation served as the means to test and evaluate the performance of TRASURF as a multi-section vehicle-monitoring algorithm. The algorithm’s ability to reconstruct individual vehicle trajectories will enable more efficient and effective traffic surveillance, and will enhance the collection and analysis of network-wide traffic information including path travel time and origin-destination matrices. Furthermore, investigations and descriptions of various applications of advanced detectors for traffic analysis, especially in the context of the single-loop configuration widely used within California and many other locations were made. Traffic data extraction based on advanced loop detectors will make a vital contribution to many aspects of traffic operations and management, as these data are not available from conventional detectors.
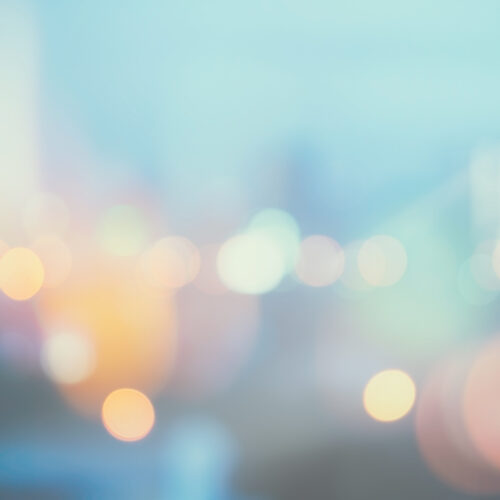
Speakers
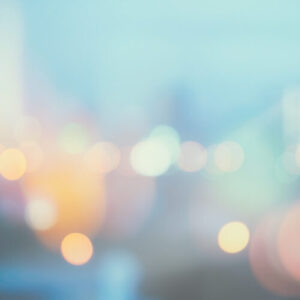
Seri Park
speaker