Events
Featured Event
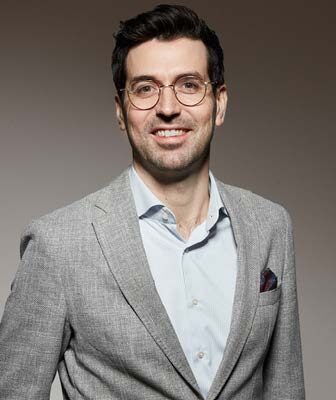
Infrastructure Assisted Cooperative and Distributed Perception Strategies for Connected Vehicles
Abstract This research addresses the challenge of efficiently processing sensor data across vehicles, edge, and cloud platforms to support resource...
Read MoreEvents
Multimodal freight transportation moves most goods across the United States, with trucks and railroads as the dominant modes. However, growing freight activity has raised concerns...
Over the last fifty years, transportation researchers have sought to develop stable day-to-day dynamical models to capture the convergence process toward a departure time user...
This dissertation uses large-scale data to analyze shared micromobility systems (SMS), such as dockless scooters and bikes. Through a combination of meta-analysis, spatial modeling, and...
Privately owned autonomous vehicles (PAVs) introduce new travel behaviors, such as remote parking, returning home, and serving other household members, potentially increasing vehicle miles traveled...
Traditional traffic control has been based on collective stop-and-go movements for over a century, but should we still hold the presumption that it is best...
Traffic Monitoring is at the center of any Intelligent Transport System and the current traffic monitoring devices are challenged to deliver in the evolving landscape...
This dissertation delves into the intersection of two critical elements shaping the future of transportation: the necessity to anticipate and explore the forthcoming transportation paradigm...
A trip chain is a series of consecutive trips to multiple destinations. By influencing activity and travel decisions, trip chaining can directly impact roadway congestion,...
This dissertation introduces a novel model for integration into an Agent-Based Model (ABM) framework, aimed at estimating and predicting workers’ commuting behaviors in a post-pandemic...
The purpose of this study is to develop a methodology for processing vehicle trajectory data which are presented as a series of discrete positions of...
This dissertation explores the evolving landscape of prepared food consumption, particularly restaurant meals, and proposes optimization strategies for managing delivery fleets. In the context of...
Cities around the world vary in terms of their transportation network structure and travel demand patterns, with implications for the viability of shared mobility services....